3 ways AI can automate customer support content
AI-generated content is the future of customer support. Mavenoid’s Galina Ryzhenko explains how to overcome its associated challenges
Add bookmarkListen to this content
Audio conversion provided by OpenAI
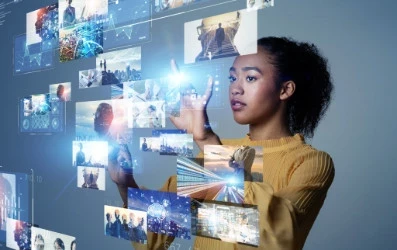
Artificial intelligence (AI) has automated many areas of business, from detecting fraudulent banking transactions to predicting consumer behavior. Applied to customer service and support, it is taking the form of virtual assistants and automated chatbots that provide consumers with a more personalized customer support experience.
It's little wonder that when CX Network asked practitioners to name their top CX trends for 2024, the 10 most selected trends included AI for operations, Generative AI chatbots and virtual assistants, Conversational AI chatbots and virtual assistants and Generative AI for marketing or hyper-personalization.
A dynamic approach to content and documentation
Traditional support content includes printed manuals and online help centers with static FAQ pages. Customer support organizations can struggle to keep this material updated as new products launch and existing products are updated.
However, this longform support documentation requires consumers to sift through large amounts of information when they try to troubleshoot, which is both time-consuming and does not guarantee they will identify the right documentation.
To solve these challenges, customer support teams have looked to new technologies like cloud-based support libraries and AI-powered chatbots. These tools offer dynamic support documentation and flows that increase self-service resolution.
In many cases, these solutions are only able to host information, but create it. Here are three examples
Generative AI case studies from customer support
1. Virtual support flow generation
Customer support teams often reference a deep repository of existing support manuals, documentation, help articles and videos. That documentation has immense value, but it is cumbersome to transform into self-service flows that consumers can use to self-troubleshoot. The process typically requires parsing large amounts of material and then organizing and editing it to be easily accessible and understood by consumers.
Large language model (LLM) technology is a form of AI that is ideal for building new support flows from large amounts of existing documentation.
An LLM digests large volumes of text and restructures it according to prompts. These models use machine learning (ML) to extract relevant information, simplify data, pinpoint knowledge gaps and generate new content. The technology can comprehend intricate queries and craft human-like responses based on the new support content.
This approach meets consumers’ preferences for remote support and can increase their self-service resolution — often up to 70 percent with AI, as opposed to the nine percent industry standard reported by Gartner in 2019.
2. Copyediting
Support organizations can input their existing support documentation into an AI program to receive suggestions for how to improve the clarity and effectiveness of their instructions.
AI may also be used to ensure correct grammar and terminology, and a consistent writing style. Finally, the technology can be used to condense dense text into support flows that take users step-by-step to their desired solution.
3. Content gap analysis and maintenance
Another way that AI helps support teams is by analyzing the effectiveness of their content. AI is well-suited for this work because it is able to analyze large amounts of information and quickly identify what is or is not working. This helps organizations answer more customer queries through self-service solutions when those queries would have otherwise been escalated to a live agent in the absence of relevant support content.
With AI, support teams can quickly generate new documentation as products are released and updated. No longer burdened by manual content management, these teams can provide a more personalized customer experience by addressing product issues regardless of whether they are being experienced by one or one hundred users.
Challenges and considerations
LLMs and generative AI are only as good as their source material, which reinforces the need for a thoughtful approach to deploying the technology. AI-generated content can contain hallucinations, incorrect or misleading information, which is often caused by biased or insufficient training source material.
To protect their brand’s reputation, organizations using AI must rely on human oversight and LLMs specifically trained to work within known parameters.
In the context of AI-enabled customer support, human oversight can be achieved through manual content checks whenever an end user might be at risk of receiving inaccurate product information. Support professionals should both leverage the speed of AI and confirm the output aligns with customers’ expectations.
Future outlook for LLMs and AI-generated content in customer support
As further development of AI continues to advance the capabilities of automated customer support, we will continue to see more use cases for applying the technology to increase business efficiency and customer satisfaction with remote support.
Though challenges like ensuring information accuracy and data safety exist, the latest advancements offer a promise of streamlining support processes to increase customer resolution and satisfaction rates, while allowing organizations to save in support costs.