The complete guide to generative AI for customer experience
CX Network’s guide to generative AI, how it benefits CX and how to implement it
Add bookmarkListen to this content
Audio conversion provided by OpenAI
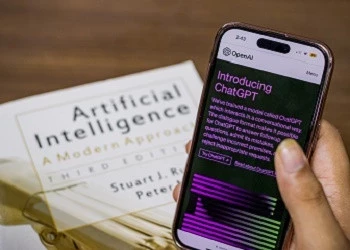
As consumer expectations evolve, organizations across industries are turning to cutting-edge technologies to stay ahead in the race for customer loyalty and market share. One of the most influential developments of recent years is generative artificial intelligence (AI), a powerful tool that is reshaping how businesses operate.
From the different applications of generative AI in CX to how to implement it along with real-world use cases, this guide is your compass for navigating this new world and its profound impact on customer experience.
Don't miss any news, updates or insider tips from CX Network by getting them delivered to your inbox. Sign up to our newsletter and join our community of experts.
Contents
- What is generative AI?
- Types of generative AI in customer experience
- The benefits for CX
- How to implement generative AI into your CX processes
- Real-world examples in CX
- Challenges and considerations
What is generative AI?
Generative AI refers to a class of artificial intelligence (AI) systems that are designed to generate content including text, images and audio in formats that resemble those that a human would produce.
These systems are trained on huge datasets and information scraped from the internet, and use machine learning (ML) techniques to generate new data. This means that by learning the underlying patterns and structures of the training data, it is able to produce new content every time it is prompted.
What is a large language model (LLM)?
By now we’ve all heard of the power of OpenAI’s ChatGPT, but it is not the only one of this powerful new class of systems also known as large language models (LLMs).
An LLM is a type of gen AI that uses deep learning techniques and vast data sets to understand, generate and predict new content.
Since the release of ChatGPT-3 in late 2022, it has been followed by Google’s Bard, Microsoft’s Bing AI and DeepMind’s Sparrow, among others (and ChatGPT is now up to version 4). These LLMs analyze and respond to user prompts in human-like language.
LLMs can also analyze images. Be My Eyes, a mobile app that connects visually impaired users with volunteers through live video calls, has introduced Virtual Volunteer, a digital visual assistant powered by GPT-4. Users upload an image into the tool, which it then evaluates so it can answer questions about it, for example describing the color of an object or the contents of a refrigerator.
How is generative AI different to conversational AI?
Conversational AI works with pre-loaded prompts to provide human-like conversation with users in natural language, for instance through chatbots and virtual assistants. Gen AI, meanwhile, is focused on creating content based on large amounts of input data.
There is some overlap between the two, as gen AI can also be used to power conversational agents by generating text-based responses in response to queries.
Examples of generative AI in customer experience
In terms of their potential to impact and transform CX as we know it, these are the main types of AI used in customer experience:
Chatbots and virtual assistants
These are advancing rapidly now due to gen AI, which enables them to produce text-based responses in natural language.
They can provide immediate responses to customer enquiries, offering support, answering frequently asked questions, scheduling appointments, and handling routine customer service interactions.
Voice assistants
Voice-activated bots use natural speech to interact with users and solve queries. Amazon’s Alexa, Apple’s Siri and Google Assistant are among the most well-known.
British fashion retailer ASOS was the first company of its kind to sell products through Enki, its fashion bot available via Google Assistant. In 2020 the company went one step further and deployed a voice assistant to work alongside frontline advisors to tackle increasing customer care workloads. This move added 50 points to its net promoter score (NPS) and saw improvements in resolution rates and waiting times.
Personalization engines
Here generative AI is used to create personalized product recommendations, content suggestions, and marketing messages based on customer behavior and preferences.
Perhaps the most famous example of this in action is Amazon’s model – it collects a vast amount of data on each user including their browsing and purchase histories as well as items they have reviewed, and uses this information to create a detailed profile for each person.
Its algorithms analyze the data and then utilize this to find items that will be of interest to the customer, making recommendations across various touchpoints including the homepage, search results and personalized emails. So next time you visit a page with “Recommended for you” at the top, that has been created with a personalized engine, a form of gen AI.
These types of generative AI are also valuable for CX:
- Visual content generation: In e-commerce and product customization, it can create visual content, such as product images or 3D product renderings, based on customer preferences.
- Voice generation: AI-powered voice generation systems create human-sounding speech for voice messages, customer service recordings and interactive voice response (IVR) systems.
- Feedback analysis: Customer feedback and survey responses can be analyzed quickly. Data is categorized automatically and insights are created from customer comments and reviews, helping businesses make data-driven decisions to improve the customer experience.
- Virtual try-on: In industries like fashion and home improvement, it is used to create virtual try-on experiences, allowing customers to visualize how products will look on them or in their homes.
- Content generation: Content is invaluable for marketing purposes, such as ad copy, social media posts, and product descriptions. An IT tool that creates content can help marketers produce engaging and relevant copy for customers (more on this below).
- Data augmentation: Behind the scenes, it can be used to augment customer datasets for training machine learning models that predict customer behavior or preferences.
- Email automation: AI-driven systems can produce automated responses to customer emails. These can turn simple text prompts into HTML.
- Social media responses: AI can also be used to automate responses to comments on social media. In the case of virtual influencers deployed by brands to boost customer engagement, the AI can produce natural-sounding responses based on specific keywords or triggers. Timely and consistent interactions help build the influencer’s following, and therefore the trust that impacts a purchase decision.
The benefits of generative AI for CX
According to a survey by Dialpad, 79 percent of sales and customer service professionals using AI tools at work have experienced a positive impact on their performance. Additionally, 84 percent said that AI tools are crucial to support their company’s growth. Here are three practical ways we have seen it already benefiting CX:
Personalization
To better understand their audience, companies need to collect customer data, but to effectively analyze it and take meaningful action requires resources that many organizations simply don’t have.
An AI is capable of analyzing vast quantities of data in far less time than a human. Andrew Carothers, senior CX leader at Cisco, says that “generative AI changes all that, allowing organizations to automate how they create and deliver recommendations, tailored responses and even proactive suggestions based on ongoing data insights. The result is a highly personalized experience – one that demonstrates that a business really cares.”
Easing the workload for contact center staff
Thanks to the large amounts of data it leverages, AI-powered chatbots and assistants can respond to simple queries in real time and faster than a human agent, freeing up their time for more complex questions and helping to reduce bottlenecks.
Michelle Martinez, formerly the head of post-order CX strategy at online furniture retailer Wayfair, explains how LLMs benefit interactions with contact center staff. “With contact centers and chatbots, a lot of information is presented to a customer.
"ChatGPT allows a more eloquent response that can potentially leverage all the information available for both agents and chatbots and can present information to customers and agents with speed and a human tone,” she says.
Enhancing content creation
Creating informative, quality content is one of the most effective ways for brands to attract and retain customers. LLMs can be great at helping you craft the outline of an article on your chosen topic, but be weary of churning out content without taking time to proof it yourself, as generic content will not get much enthusiasm from customers.
CX expert and keynote speaker Dan Gingiss expands on this: “ChatGPT can do a great job of building a template for a blog or building an outline for a blog in which you then fill in some of the details. What I have found is that on a lot of topics it is very good at scratching the surface, but it is less good as you want to get into the real nitty gritty details. Again, I think it will get better at that over time.
Gingiss adds: “I would not be using it to pump out automated content, but I definitely think it can be used as a starting point for that, especially as more and more technologies are coming out of ChatGPT.”
How to implement generative AI into your CX processes
All the benefits listed above are linked by one vital component: data. An AI will only be as reliable as the data it is fed (hence the term, ‘garbage in, garbage out’), therefore understanding, preparing and using data insights effectively with adequate safeguards in place is crucial when it comes to adopting AI.
Given that developing an AI in-house is resource-heavy and requires a great level of expertise, most organizations will partner with solutions providers to use existing models. However these steps should be taken into account when adopting this technology:
1. Define your objectives
Start by identifying the specific CX goals you want to achieve. Do you want to create a more personalized customer journey? Speed up response times? Automate repetitive tasks to free up agents’ time, or something else? This will help you decide what AI tool to use.
2. Select the right solution
Consider how complex your tasks are, the scale of your organization and what you want to achieve before choosing a solution.
3. Understand and prepare the data you are capturing
Data can come from CRM systems, chat logs, surveys and social media, among other sources. Make sure it is relevant to your objectives. Before it is fed to the AI it may need to be cleaned and labelled (this is typically done by the engineer/data scientist).
4. Train the AI
This is a crucial step for adapting the model to your specific processes, language and context. It involves adjusting the model’s parameters and then monitoring different metrics to ensure it is accurate. AI training is usually performed by engineers as it is a complex task and requires a fair amount of fine-tuning.
5. Integrate it with CX channels
To ensure the AI is seamlessly integrated with chatbots, virtual assistants, email responses or any other CX channels, engineers will write coding and perform testing to ensure there are no errors.
6. Make sure you are compliant with data protection laws
Ensure you are complying with relevant data privacy regulations, such as the General Data Protection Regulation (GDPR) in Europe, or the California Consumer Privacy Act (CCPA) in the US. Implement safeguards to protect customer data and be transparent with customers about data usage. There is more on this in the section below.
7. Get feedback on the new processes
Once up and running, continuously collect feedback from customers and agents to find areas for improvement, tweaking the AI where needed.
8. Train your human agents
Human agents will need training and support to work alongside the tool. They should understand how to collaborate effectively with the AI and step in when necessary.
9. Measure ROI
Evaluate the return on investment (ROI) of the implementation. To do this, assess things like customer satisfaction and response times to measure the impact on your CX processes.
Real-world examples in CX
For organizations looking to adopt an AI solution, there is no shortage of innovative tools on the market. Here are just a few that caught our attention:
Twilio’s customer journey mapping tool
Marketers will soon be able to skip the manual process of architecting customer journeys thanks to the new CustomerAI Generative Journeys tool developed by Twilio. It will enable users to describe campaign type, define their audience and which channels they want to use. Twilio then automatically builds the customer journey.
EvaluAgent’s quality assurance tool
EvaluAgent has created Auto-QA for quality assurance in the contact center.
Using AI-driven analysis, it identifies important moments within conversations, and detects and redacts sensitive customer data. It also generates improvement suggestions, summarizes conversations in bullet points, and uses data to identify conversations requiring urgent attention.
eBay’s new function for sellers
Earlier this year eBay launched a plug-in that uses gen AI to automatically create text for items that sellers want to list, helping to cut down on the time and effort it takes to make a listing. It can also leverage insights from the 20 billion images it has stored in its index, helping sellers to create better product images.
Duolingo's responsive language learning
Language-learning platform Duolingo is using ChatGPT-4 to help users practice conversational skills. The feature then offers AI-powered feedback on the accuracy of responses to explain where learners went wrong, so they can continuously improve.
Read more about brands like Coca-Cola and Expedia using AI in 7 ways companies are using generative AI in customer experience.
Challenges and considerations
As with all nascent technology, organizations must take concerns around data privacy, transparency and trust into consideration to avoid being on the wrong side of the law and alienating customers.
Data concerns
A concern that frequently arises among customers is how their personal data is used by AI platforms. There have been incidents such as the case of ScatterLab in South Korea, where data from 10 billion conversation logs, originally collected for a dating counselling service, were used to develop an AI chatbot called Lee-Luda.
Unfortunately weeks after it launched the chatbot exposed sensitive information, and even used abusive and sexually explicit comments in interactions with customers. This privacy breach resulted in a US$93,000 fine by the South Korean government.
To avoid this, organizations must prioritize transparency and data privacy, adhering to regulations.
Compliance
If all goes to plan, by the end of 2023 AI will be regulated in the European Union by the AI Act, the world’s first comprehensive AI law. This will naturally have implications for companies operating within the region as well as those that do business with the bloc. Once the act is in place, organizations using AI will have to comply with these transparency requirements:
- Disclosing content that has been generated by AI
- Designing the model to prevent it from generating illegal content
- Publishing summaries of copyrighted data used for training
In the US, draft legislation is in the early stages, however the White House has announced that it has reached a voluntary agreement with seven prominent generative AI companies to provide minimum guardrails for safety, security and public trust.
Balancing automation with the human touch
According to research by SurveyMonkey, 90 percent of the public prefer customer service from a human rather than an automated chatbot. While AI has clear benefits in terms of speed and efficiency, it cannot replace the emotional intelligence and empathy that are necessary to build customer loyalty.
Chandni Bhatt, senior UK manager, member happiness at Beauty Pie, explains that to maintain a human element while deploying a chatbot for customer queries, her team wrote out all the responses to ensure consistency with branding and language. And rather than issue instant responses, queries are answered within five minutes, so the bot seems as human as possible.
“We steer away from even calling it a bot,” Bhatt told us. “We wanted to keep that human element, so we made sure we mapped out everything that we could, to see what we can automate and what we absolutely cannot, taking the team on the journey and making sure complex queries remain with our agents.”
Getting the balance right between automation and human intervention is something of an artform. Customer-centric businesses need to master this to make the best use of the strengths of both the AI and the human agents.
All Access: Voice of the Customer 2025
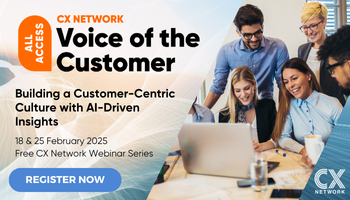
Join us to hear from industry leaders, innovators, and CX experts as they share insights, strategies, and tools for harnessing customer feedback to drive meaningful change.
Register Now